Decoding the 2024 Nobel Prize in Physics: The Neural Network Discovery That Defined The Artificial Intelligence (AI) Era
The 2024 Nobel Prize in physics was awarded to Professor Emeritus John Hopfield from Princeton University, USA and Professor Emeritus Geoffrey Hinton from the University of Toronto, Canada for their pivotal contributions to artificial neural networks (ANNs). Their work has significantly influenced machine learning and laid the groundwork for the modern AI era. Professor Masayuki Ohzeki from the Institute of Science Tokyo offers insights into their accomplishments.
What is the reason behind the 2024 Nobel Prize in physics?
Ohzeki The decision to award the Nobel Prize in physics for advancements in AI may seem surprising. However, the foundations of ANNs are deeply rooted in principles derived from physics. Before discussing their contributions in detail, let me first explain what machine learning is and how it works.
Machine learning enables computers to identify patterns in data, make predictions, and perform specific tasks. This technology is powered by ANNs, which are inspired by the structure of the human brain.
In the human brain, neurons transmit signals to one another thereby forming intricate connections, which are strengthened through repeated exposure. For example, when we repeatedly practice solving arithmetic problems, the connections between our neurons become stronger, helping us to recall and solve similar problems more easily with time.
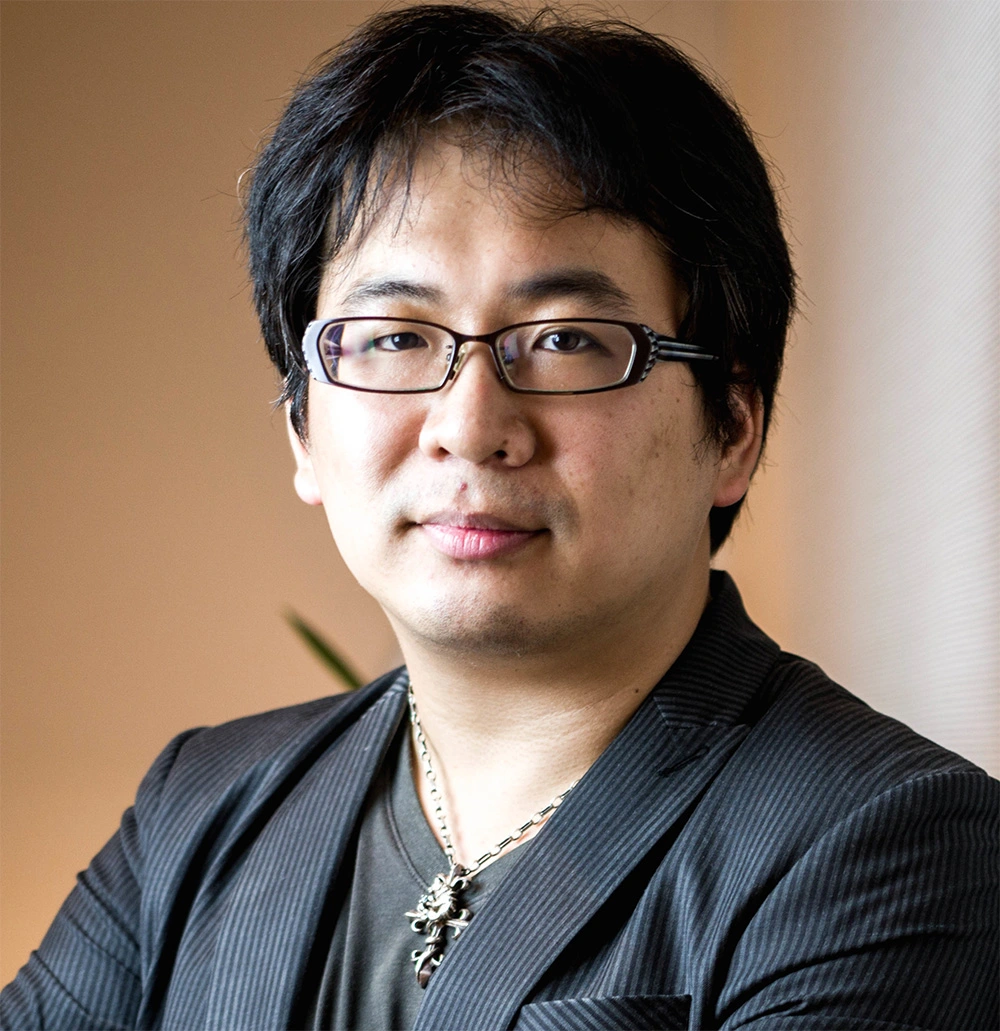
In ANNs, neurons are represented by nodes, and signals are exchanged between them. These signals are assigned weightage to determine their importance. Through repeated adjustments over time, the network learns to make more accurate decisions. This learning ability enables neural networks to improve their performance by analyzing data.
Professors Hopfield and Hinton independently proposed strategies for developing machine learning through ANNs. Their pioneering work laid the foundation for today’s AI technologies, including voice and image recognition, as well as self-driving cars.
What kind of research did Professors Hopfield and Hinton conduct on machine learning with ANNs?
Ohzeki The development of ANNs began when the focus of computing shifted from time-consuming calculations to recognizing complex patterns in data.
The first significant advancements in this field were made in 1943 by Warren McCulloch and Walter Pitts, who introduced the concept of artificial neurons modeled after the human brain. Although these early models laid the groundwork for neural networks, they had limited learning capabilities and could only solve basic problems.
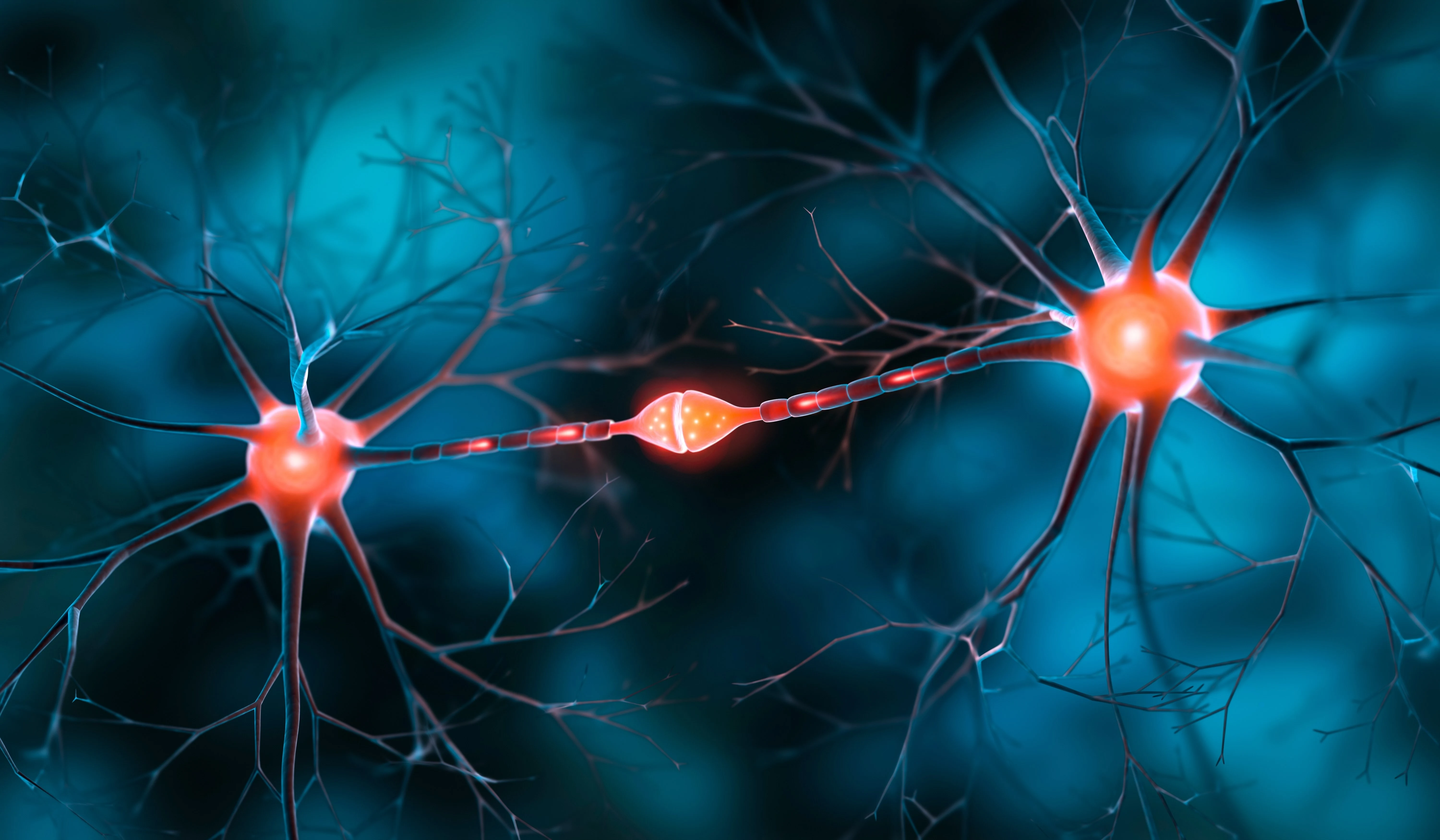
The networked structure of ANNs, as we know them today, emerged in the 1980s when researchers speculated that neurons could exhibit collective behavior, similar to humans in a crowd with a common intention.
This idea led to important breakthroughs by Professors Hopfield and Hinton, who were inspired by "spin glass theory," a concept from physics.
Spin glasses are systems where atomic spins—a type of rotational motion, similar to a figure skater spinning—are randomly oriented but remain fixed in their places. These systems typically settle into states of minimum energy when some pairs of spins align in the same direction, forming magnetic domains. If a disturbance flips some spins within the domain, the system naturally returns to a stable state over time, minimizing its energy.
In 1982, John Hopfield, a theoretical physicist and biologist, applied this idea to artificial neurons by introducing the "Hopfield Network." In this network, neurons are represented as binary nodes, and their state—either active (on) or inactive (off)—analogous to the direction of the spin.
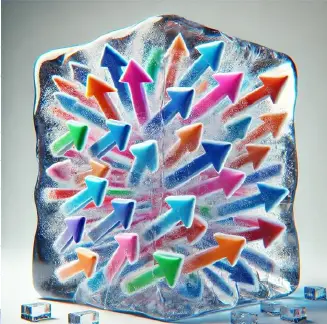
The Hopfield Network exhibits stationary states representing stored patterns that the network can "remember." When presented with an input resembling one of these stored patterns, even if it is distorted or incomplete, the network adjusts the states of individual nodes to settle into the closest match among its stored patterns. Prof. Hopfield's work revolutionized ANNs by demonstrating the strategy by which they could remember patterns and recognize distorted or incomplete data.
In 1985, Geoffrey Hinton, a British computer scientist, expanded on the Hopfield Network to develop a new type of ANN known as the Boltzmann Machine. It employs a statistical approach to recognize patterns directly from the data itself. This allows for more flexible learning and classification of data, and creation of new models based on the recognized pattern.
Initially, the Boltzmann Machine required significant computational resources, which made its practical implementation challenging. However, through continuous refinement, Hinton and his team developed more efficient network structures and learning algorithms.
These breakthroughs laid the groundwork for the rise of deep learning in the 2000s, enabling the creation of generative models capable of producing advanced text, images, videos, and music.
How does your research at Science Tokyo relate to their achievements?
Ohzeki My research focuses on quantum computing, particularly using spin glass theory. In spin glass systems, atomic spins can be in two states, similar to the 0 and 1 bits in computers. This theory can be applied to solve many types of computational problems. Quantum computing is a multidisciplinary field which utilizes complex concepts from mathematics and physics to solve challenging problems. While studying at Tokyo Institute of Technology (now Institute of Science Tokyo), I studied quantum annealing under the guidance of my mentor Professor Hidetoshi Nishimori, who developed its core theory[related article]. Quantum annealing is one of the first practical techniques which optimizes the processes in quantum computing.
At Science Tokyo, we are also exploring "quantum Hopfield models," which combine Hopfield networks with quantum mechanics. If you are interested in AI or quantum computing, I invite you to join us to help advance this exciting field.
Related article
*This article is based on the content of the Science Tokyo Nobel Prize Lecture held online on Wednesday, November 20, 2024.
Discover More About Professor Ohzeki's Work
Ongoing Similar Research at Science Tokyo
Contact
Research Support Service Desk
- Remarks
- For inquiries, please contact us at https://www.rdc.isct.ac.jp/en/contact-us/.