Generative AI masters the art of scent creation
A novel model using generative diffusion neural network automatically creates custom fragrance recipes, based on user input of scent descriptors
Addressing the challenges of fragrance design, researchers at Institute of Science Tokyo (Science Tokyo) have developed an AI model that can automate the creation of new fragrances based on user-defined scent descriptors. The model uses mass spectrometry profiles of essential oils and corresponding odor descriptors to generate essential oil blends for new scents. This breakthrough is a game-changer for the fragrance industry, moving beyond trial-and-error, enabling rapid and scalable fragrance production.
AI-Driven Aroma Design: Creating New Fragrances Using Diffusion Network
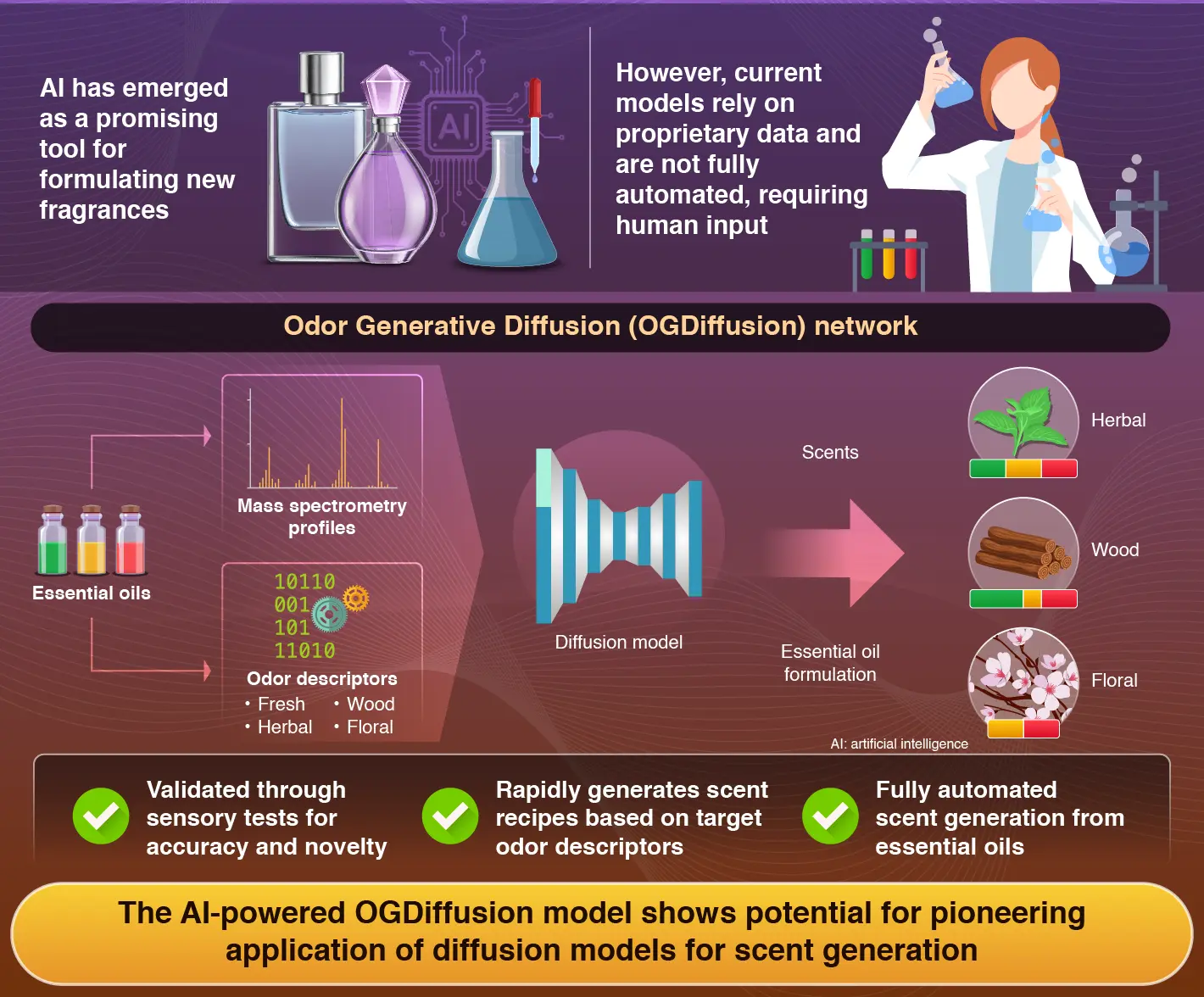
Aleixandre et al. (2025) | IEEE Access
Designing new fragrances is crucial in industries like perfumery, food, and home products, where scent significantly influences the overall experience of these products. However, traditional fragrance creation can be time-consuming and often depends on the skill and expertise of specialized perfumers. The process is typically challenging and labor-intensive, requiring numerous trial-and-error attempts to achieve the desired scent.
To automate this process, a research team, led by Professor Takamichi Nakamoto from Science Tokyo, developed an AI model called Odor Generative Diffusion (OGDiffusion). This model utilizes generative diffusion networks, a type of machine learning model that learns to create new content by reversing a noise process informed by existing data. These models are already widely employed to generate images and text, and the team has adapted this technology to create new fragrances. Their findings were published in IEEE Access on March 27, 2025.
The system operates by analyzing the chemical profiles (mass spectrometry data) of 166 essential oils, which are labeled with nine odor descriptors (such as "citrus" or "woody"). When users specify desired scent characteristics, AI generates a corresponding chemical profile (mass spectrum) that aligns with those descriptors. It then calculates the mix of essential oils needed to recreate that scent using a mathematical method called non-negative least squares.
"Our diffusion network uses patterns in mass spectrometry data of essential oils to generate new fragrance profiles in a fully automated, streamlined, and data-driven approach while maintaining high-quality data output. By eliminating human intervention and molecular synthesis from the process, we provide a fast, general, and efficient method for fragrance generation," explains Nakamoto.
While existing AI-based fragrance generation models, they have been developed, they rely on proprietary datasets and still require expert input. The primary advantage of the new method is its ability to automate the creation of new scents completely. Moreover, as the system produces fragrances based on essential oil recipes, the final scent can be easily recreated.
Further, the team conducted human sensory tests to evaluate whether the AI-generated fragrances align with the intended scent profiles. In a double-blind setup, 14 participants were tasked with matching AI-generated fragrances to appropriate descriptors (such as "citrusy" or "floral"). Participants were consistently able to identify the correct fragrance, demonstrating that the system could produce scents that met people's expectations. In another test, participants distinguished between two scents: one designed to express an additional specific odor descriptor and original scent without that descriptor. They reliably selected the scent that matched the target descriptor, indicating that the model generates clear and identifiable scent profiles.
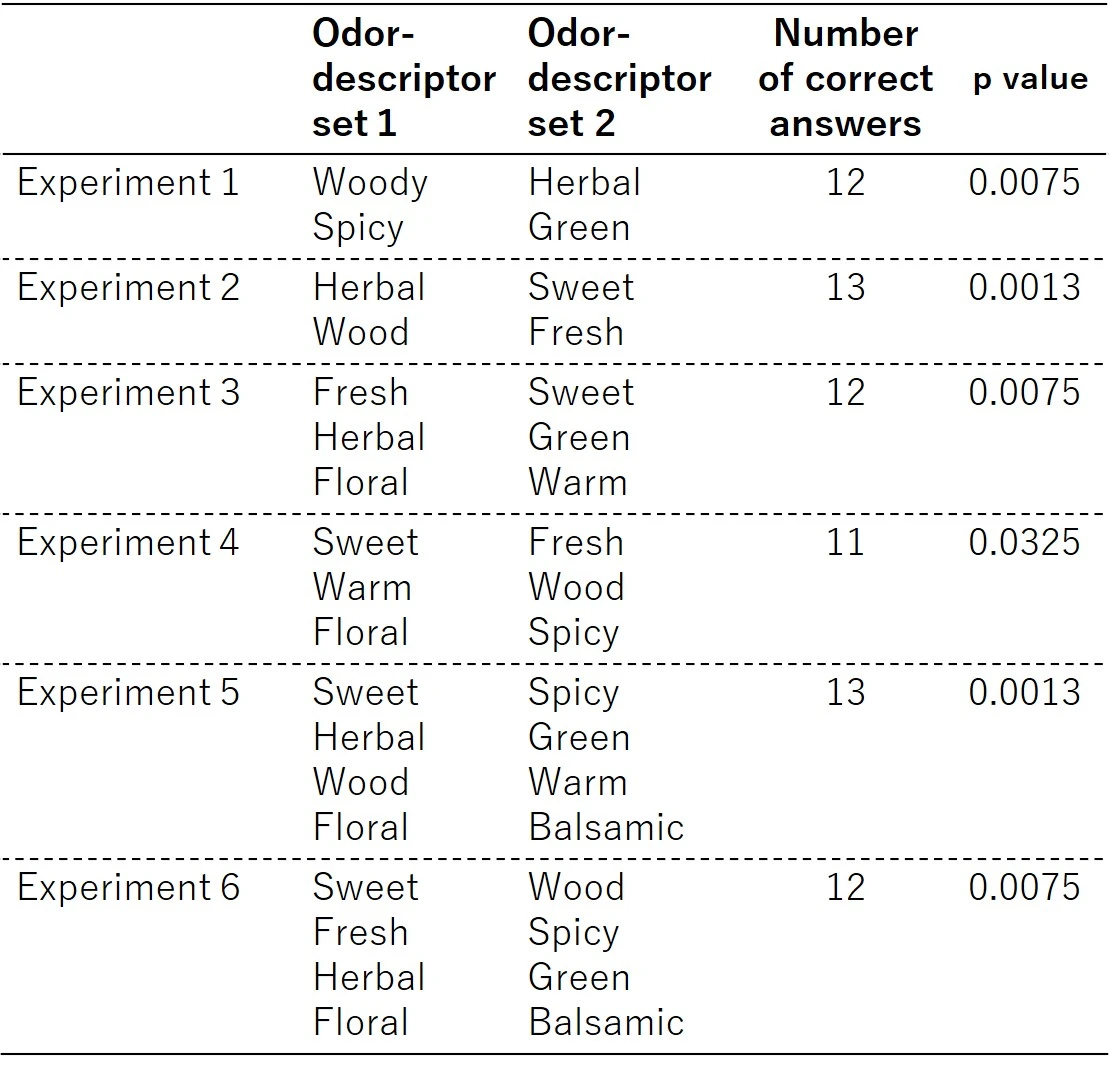
Results of the sensory test with 14 assessors. This test examined whether participants could classify the generated scents according to the provided sets of odor descriptors. The number of correct answers indicates how many participants correctly identified each intended scent. We can say that the participants correctly classified the intended scents under the significance level of 5% since all p-values were below that level.
Nakamoto’s model—the first of its kind—heralds a future in which AI transforms scent design. “This approach represents a significant advancement in aroma design,” states Nakamoto. Adding further, he says, “By automating the generation of mass spectra corresponding to desired odor profiles, the OGDiffusion network offers a more efficient and scalable method for fragrance creation. Moreover, even a novice can create an intended scent to make scented digital contents”
In summary, this innovative method allows for faster and more flexible scent design, with potential applications across various industries. By leveraging AI for scent generation, the OGDiffusion model demonstrates that computers can indeed possess a nose for creativity.
Reference
- Authors:
- Manuel Aleixandre, Dani Prasetyawan, and Takamichi Nakamoto*
*Corresponding author - Title:
- Generative Diffusion Network for Creating Scents
- Journal:
- IEEE Access
- Affiliations:
- Laboratory for Future Interdisciplinary Research of Science and Technology (FIRST), Institute of Innovative Research (IIR), Institute of Science Tokyo, Japan
Related articles
Further Information
Professor Takamichi Nakamoto
Laboratory for Future Interdisciplinary Research of Science and Technology (FIRST), Institute of Innovative Research (IIR), Institute of Science Tokyo, Japan
Contact
Public Relations Division, Institute of Science Tokyo
- Tel
- +81-3-5734-2975
- media@adm.isct.ac.jp